Maximizing Efficiency with Machine Learning Labeling Tools
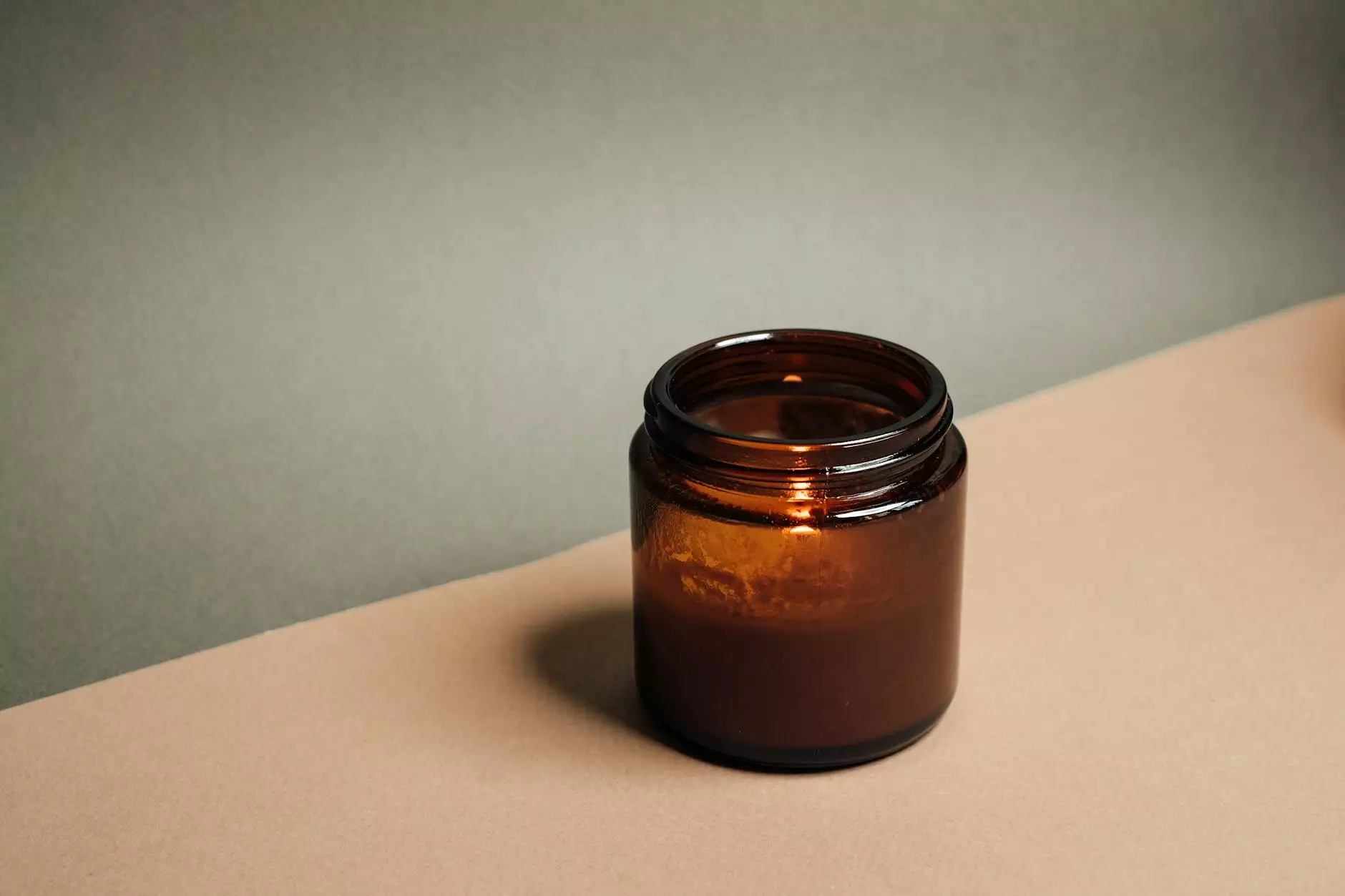
In today’s fast-paced technological landscape, machine learning labeling tools are emerging as essential components that enable businesses to harness the power of data. These tools are at the forefront of data annotation technology, transforming raw data into structured formats that machine learning algorithms can effectively utilize. This article explores the significance of these tools, their applications, and how they can substantially benefit businesses, particularly in the realm of data science and artificial intelligence.
Understanding Machine Learning Labeling Tools
Machine learning labeling tools are software applications designed to assist in the process of labeling or annotating data. This process is pivotal in the training phase of machine learning models, wherein algorithms learn from labeled data to make predictions or classifications. Properly labeled data leads to enhanced learning outcomes, improved accuracy of models, and ultimately better decision-making processes.
The Importance of Data Annotation
Data annotation refers to the method of tagging or labeling data sets so that machine learning models can interpret them. The quality of data annotation directly influences the performance of machine learning solutions. Effective labeling is critical for:
- Supervised Learning: In supervised learning, models learn from labeled data. The accuracy of predictions hinges on correct annotations.
- Quality Control: Comprehensive and accurate labeling ensures that the data used for training is reliable, thus reducing errors in model outcomes.
- Efficiency Enhancements: Automated labeling tools can significantly reduce manual effort and increase the speed of data processing.
Key Features of Machine Learning Labeling Tools
When considering a machine learning labeling tool, there are several key features to look for that will improve productivity and ensure data quality:
1. User-Friendly Interface
A user-friendly interface allows team members, regardless of their technical expertise, to navigate the labeling tool with ease. This accessibility promotes broader use within organizations and accelerates the annotation process.
2. Support for Multiple Data Types
Effective labeling tools accommodate various data types, including images, texts, audio, and videos. The ability to manage different types of data efficiently is crucial for versatile machine learning applications.
3. Collaboration Features
Collaboration is vital in any project involving multiple stakeholders. Tools that offer real-time collaboration allow teams to work together seamlessly, ensuring consistency and reducing the risk of errors.
4. Quality Control Mechanisms
Implementing quality control measures, such as verification processes or consensus mechanisms, helps maintain high standards of data accuracy and minimizes the discrepancies in labels.
5. Integration with Existing Workflows
Machine learning labeling tools should integrate smoothly with the organization's existing data workflows. This integration enhances functionality and minimizes disruptions during data processing.
Benefits of Using Machine Learning Labeling Tools
The adoption of a machine learning labeling tool confers numerous advantages across different industries:
1. Increased Efficiency
By automating the data labeling process, organizations can drastically reduce the time spent annotating data, allowing data scientists and engineers to focus on model development and fine-tuning rather than manual tasks.
2. Enhanced Data Quality
These tools are designed to minimize human error through automation and provide sophisticated algorithms that can standardize labeling practices. This ensures higher data quality, which is crucial for effective machine learning.
3. Scalability
As the volume of data continues to grow exponentially, machine learning labeling tools allow businesses to scale their annotation processes without sacrificing quality or adding significant workloads on human resources.
4. Better Model Performance
The accuracy of machine learning models is directly tied to the quality of labeled data. By leveraging high-quality machine learning labeling tools, organizations can achieve better performance from their AI systems, ensuring more accurate predictions and insights.
How Key Labs Innovates in Data Annotation
At Key Labs, we understand the challenges associated with data annotation and the need for reliable solutions. Our Data Annotation Tool and Data Annotation Platform are specifically designed to address these challenges by providing cutting-edge features that enhance productivity and accuracy:
Comprehensive Annotation Solutions
Our platform supports a variety of annotation types, including image segmentation, object detection, and text categorization. This flexibility ensures that no matter the data type, we have the tools you need to succeed.
AI-Powered Automation
Utilizing advanced algorithms, our machine learning labeling tools automate rote tasks while allowing skilled annotators to focus on more complex decision-making areas. This not only speeds up the process but also optimizes resource allocation.
Robust Data Security
We prioritize data privacy and security at Key Labs. Our platforms comply with global data protection regulations, ensuring that your sensitive information is safeguarded.
Case Studies: Success Stories with Machine Learning Labeling Tools
Several organizations have successfully leveraged machine learning labeling tools from Key Labs to enhance their operations:
1. Healthcare Sector
A leading healthcare provider utilized our labeling tools to annotate medical imaging data for training diagnostic models. The rapid annotation process allowed the company to accelerate research timelines, leading to quicker, data-driven decisions in patient care.
2. Retail Industry
A prominent retail chain implemented our solutions to analyze customer feedback and product reviews. By accurately labeling sentiments, the company gained insights into customer preferences, which significantly boosted product placement strategies and marketing campaigns.
3. Autonomous Vehicles
In the autonomous vehicles sector, a major player adopted labeling tools to annotate vast datasets of driving images. This meticulous labeling process was crucial for training models that enhance driver safety and navigation systems.
Future Trends in Machine Learning Labeling Tools
The landscape of machine learning labeling tools is evolving rapidly. Here are some future trends to watch:
1. Advances in Natural Language Processing (NLP)
As NLP technologies advance, tools for text data annotation will become more sophisticated, enabling deeper analysis and understanding of textual data.
2. Increased Use of AI in Annotation
AI is expected to play a larger role in automating the labeling process, making it faster and more reliable, all while minimizing human oversight.
3. Development of Self-Learning Algorithms
Future labeling tools may incorporate self-learning capabilities that allow them to improve over time, learning from the annotations they have processed previously.
Conclusion
In conclusion, the role of machine learning labeling tools in the data annotation landscape cannot be overstated. They enhance efficiency, ensure data quality, and unlock the true potential of machine learning models. At Key Labs, we are committed to providing innovative solutions that cater to the evolving needs of businesses across various sectors.
As organizations strive to remain competitive in an increasingly data-driven world, adopting the right data annotation tool is crucial. Explore our offerings at Key Labs and harness the power of accurate data labeling to drive your business forward.